Why Dynamic Programming Is Not Good Enough
Date:
Tuesday, 7 February 2017
Time: 10.30am – 12.00pm
Speaker: Prof. Cao Xi-ren
Venue: I³ Building, 21 Heng Mui Keng Terrace, Executive Seminar Room, Level 4
Why Dynamic Programming Is Not Good Enough
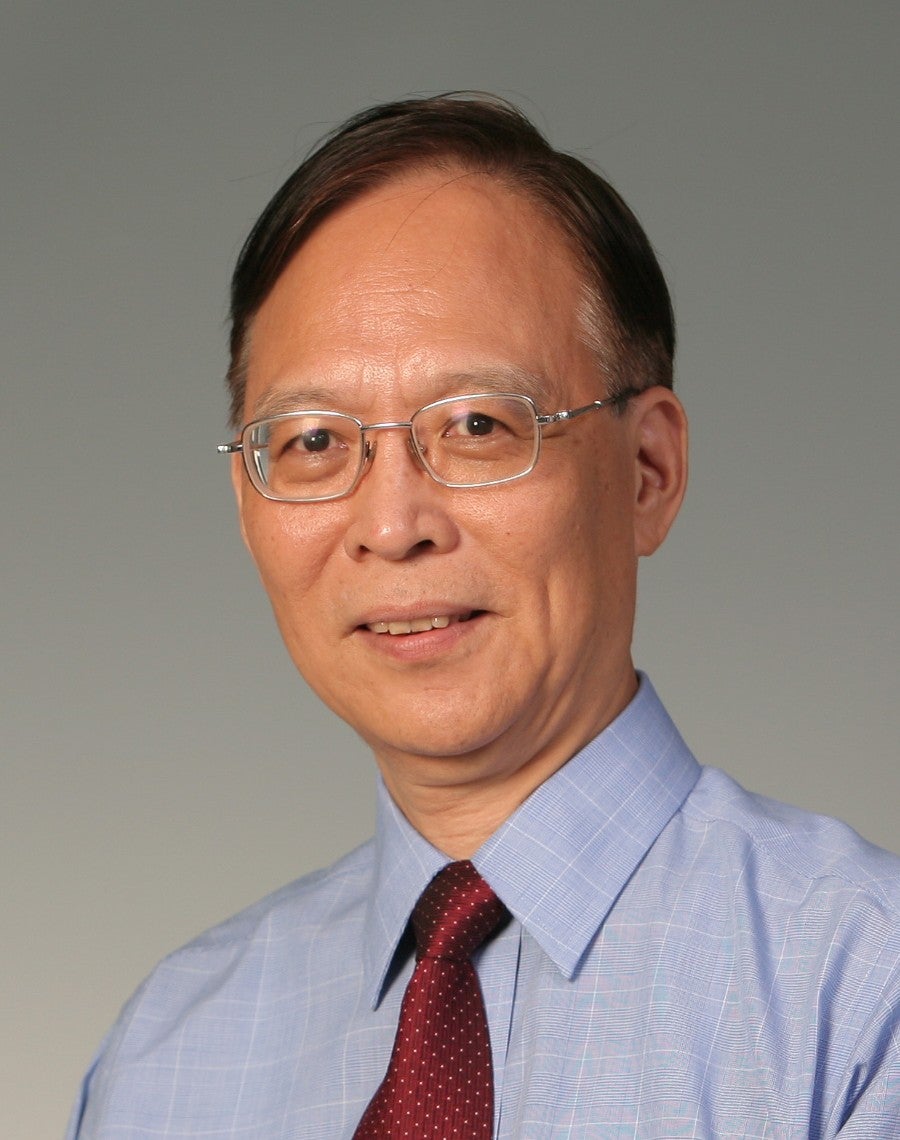
Prof. Cao Xi-ren
Shanghai Jiao Tong University and Hong Kong University of Science and Technology
About the Speaker
Cao Xi-ren is now a Chair Professor of Shanghai Jiao Tong University. He holds a PhD degree from Harvard University.
He has worked as a consulting engineer for Digital Equipment Corporation, U.S.A, a research fellow at Harvard University, as well as a reader, Professor, and Chair Professor at HKUST. He owns three patents in data- and tele- communications and has published three books in the areas of performance optimization and discrete event dynamic systems. Selected honors include being Fellow of IEEE and IFAC and best paper awards from the IEEE Control Systems Society and the Institution of Management Science, U.S.A, and a National Science Award (2nd class), China. He was the Editor-in-Chief of Discrete Event Dynamic Systems: Theory and Applications, and has served as Associate Editor at Large of the IEEE Transactions of Automatic Control, and as Member on the Technical Board of IFAC.
His current research areas include stochastic control, financial engineering, stochastic learning and optimization, and discrete event dynamic systems.
About the Seminar
With its intrinsic weakness, dynamic programming fails to address many problems. Dynamic programming works backwards in time and only provides local information at time t and in the neighborhood of x; resulting in a differential equation at (t,x). It cannot solve, for example, the under selective issue of long-run average; i.e., the optimal policy does not depend on the actions in any finite period. When the value function is not smooth, viscosity solution is used, and this hinders the development of further research due to lack of insights, as a result, degenerate diffusions are not well investigated.
We introduce another approach called relative optimization, which is based on a direct comparison of the performance measures under any two policies, and it provides global information to the optimization problem. With this approach, optimality conditions that take under selectivity into consideration can be derived; it is clear that for long-run average performance, the effect of non-smooth value function can be simply ignored; for finite horizon problems and optimal stopping, optimality condition can be derived at the non-smooth points of the value function, and viscosity solution is not needed; and the effect of degenerate points can be investigated; it is shown that they divide the state space into multi-classes and multi-class control problems can be formulated and solved.
For more information on all our workshops & seminars, please visit Risk Management Institute website.
For enquiries, please contact Nicole Wang at rminwc@nus.edu.sg
Copyright 2006-2017 © NUS Risk Management Institute